Forestry
Geographic Information System (GIS) and Light Detection and Ranging (LiDAR) technology are being used in forest management. GIS is an essential tool for optimizing current working methods in forest management. Conventional ground-based surveys for assessing forest structures can be time consuming, while LiDAR remote sensing provides a more efficient alternative. LiDAR is an advanced technology that estimates the 3-dimensional structure of forest vegetation. LiDAR data is a point cloud dataset that can be used to generate 3D maps and models. This document provides the basics of LiDAR technology and its applications in forestry. Briefly, it explains how LiDAR works, different platforms for collecting LiDAR data, and sources for LiDAR data.
What Is LiDAR?
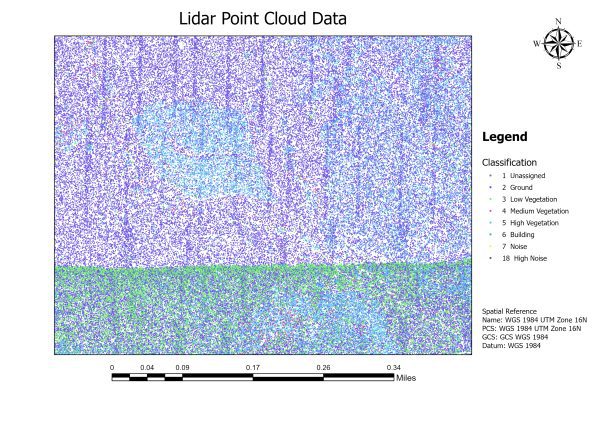
Figure 1. LiDAR point cloud data of Mary Olive Thomas Demonstration Forest in Auburn, Alabama, illustrating the 2D distribution of points captured by LiDAR technology (data source: OpenTopography).
LiDAR, also called 3D laser scanning, is an active remote sensing system that employs laser signals to determine distances based on the time delay of the returned laser pulses. The emitted pulses travel to the ground, reflecting off objects such as buildings and tree branches. The reflected laser pulses are then captured by the LiDAR sensor and recorded. By measuring the time it takes for the emitted laser pulses to travel to the ground and back, the LiDAR system calculates the distance traveled. The distance traveled is then converted into elevation. The LiDAR system employs key components, such as a GPS, to determine the X, Y, Z location and an inertial measurement unit (IMU) that provides the platform’s orientation containing the sensor. By utilizing these parameters, it is possible to calculate the 3D position of the target object. The storage of LiDAR data typically involves using file formats such as .las and .laz. The .las and .laz file formats have high compression ratios, reducing the data storage space required. These file formats also support georeferencing, which is crucial for spatial analysis. Moreover, .las and .laz formats can store various types of metadata, such as point classification and color information, which can be used for further analysis.
Examples of LiDAR Applications in Forestry
- Terrain and geomorphological applications: Forest planning and management and creating digital elevation products (digital surface model [DSM], digital terrain model [DTM]).
- Forest inventory: Provide detailed information on forest structures, including tree height, diameter, volume, leaf area index, etc.
- Forest fire management: Monitor forest fire patterns, information on forest fuel load, etc.
- Forest restoration: Identify degraded forest areas and plan forest restoration activities.
LiDAR Platforms
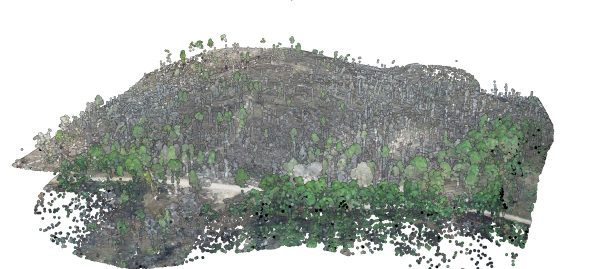
Figure 2. LiDAR point cloud data of a tornado-damaged forest area.
LiDAR platforms refer to the various systems used to carry out LiDAR sensors. Various types of platforms to collect LiDAR data are described below.
- Spaceborne LiDAR. A LiDAR system based on satellites detects 3D information about the earth’s surface with high precision and near real-time capability. This system employs a satellite platform with a photon-counting LiDAR, the primary payload. The LiDAR system collects 3D point clouds simultaneously across the mission area.
- Airborne LiDAR. Airborne LiDAR is usually acquired by manned aircraft; it can cover large ranges of areas, but it requires a significant budget and infrastructure. Figure 1 shows a two-dimensional representation of LiDAR point cloud data collected from a manned aircraft.
- UAV-borne LiDAR. Unmanned aerial vehicle (UAV)–borne LiDAR is used when a drone carries a LiDAR system and captures data. It typically consists of a LiDAR, GNSS (global navigation satellite system) receiver, and IMU to determine the orientation and speed of the LiDAR payload (figure 2).
- Terrestrial LiDAR. Mobile LiDAR and static LiDAR are the two main types of terrestrial LiDAR. The mobile acquisition involves mounting the LiDAR system on a moving vehicle. Static acquisition involves mounting the LiDAR system on a tripod or stationary device. Both LiDAR sensors consist of eye-safe lasers.
Things to Consider When Selecting LiDAR Platforms
Cost
Using UAV-borne LiDAR has become a cost-effective and versatile option for mapping small areas. While airborne LiDAR can map vast regions, monitoring and mapping large areas using this technology still pose significant challenges because of the logistical requirements, high costs, and enormous amount of data involved. UAV-borne, spaceborne, and airborne LiDAR offer cost and time advantages over terrestrial LiDAR. It can cover more area in a shorter period and does not have to navigate obstacles such as trees or power lines. Terrestrial LiDAR is typically used for more detailed examinations of specific objects and in areas where airborne LiDAR is not feasible. For example, terrestrial LiDAR can map geological features, map complex urban environments, such as narrow streets or densely built areas, or survey the interiors of buildings or infrastructure where airborne LiDAR cannot provide sufficient resolution or coverage. In forestry, terrestrial LiDAR is commonly used for plot-level forest inventories, species identification from bark structure analysis, canopy cover, gap fraction assessment, etc.
Precision and Accuracy
The precision of LiDAR data is determined by various factors, including the quality of the LiDAR system and sensor, calibration settings of the LiDAR system components, and parameters used during data collection. The accuracy of the data reflects how closely the measurement aligns with its actual value.
Data Resolution
The concept of data resolution in LiDAR refers to the density of points captured by the system within a given area. Using UAV-borne LiDAR can provide a more detailed representation of the surface being mapped compared to other aerial options, such as helicopters and airplanes, as it allows for data collection at lower altitudes and slower speeds, resulting in a higher number of points per square meter. This feature of UAV LiDAR makes it particularly useful for capturing highly detailed information about specific areas.
Sources for LiDAR Data
LiDAR data can be obtained from multiple sources, both publicly accessible and private, either free or at a cost. When obtaining LiDAR data, it is crucial to consider various specifications such as format, projection, datum, classification, and adaptability or usability of various sources. These specifications can affect the usability of the data and its compatibility with various tools and software.
There are numerous sources of LiDAR data available, including the following:
- USGS Earth Explorer
- NOAA’s Digital Coast Data Access Viewer
- Open Topography
- National Ecological Observatory Network (NEON)
- US Interagency Elevation Inventory
Many local, state, and federal agencies also provide LiDAR data online.
Another option for acquiring LiDAR data is to collect it using a drone and LiDAR sensor. LiDAR data acquisition using drones offers several advantages, such as a more detailed representation of the surface being mapped. However, there are limitations: the laser scanner’s range is limited, and the data acquisition rate is lower than airborne LiDAR systems. The accuracy of drone GPS and attitude sensors can also affect data quality. The drone’s flight time and payload capacity limit the coverage area and the type of sensor used. Several factors should be considered when generating LiDAR data with drones. These include the drone’s stability, payload capacity, and flight time; the accuracy of the GPS and attitude sensors; the laser scanner’s quality and range; and data processing methods. Using GCPs and considering vegetation density and topography during flight planning can also improve data accuracy.
It is crucial to consider various specifications such as format, projection, datum, classification, and adaptability or usability of various LiDAR data sources because these factors affect the data’s quality, accuracy, and usefulness. For example, data format and projection can affect the ability to analyze and visualize the data in different software programs, while datum affects the accuracy of the data to the earth’s surface. Classification can affect the ability to differentiate between various point cloud features, such as vegetation or buildings, and adaptability or usability refers to the suitability of the data for a particular application or research question. By considering these factors, users can ensure that the LiDAR data they obtain is appropriate for their intended use and will provide accurate and reliable results.
Software and Tools for Analyzing LiDAR Data
The analysis of LiDAR data involves several key steps, including preprocessing, visualization, and postprocessing. Various software and tools are available for preprocessing, visualizing, and postprocessing data, each with unique features and capabilities. The choice of software will depend on factors such as cost, project requirements, desired output, size of the data, and available computing power. Preprocessing involves preparing the LiDAR point cloud data by removing outliers, classifying ground points, and eliminating errors and nontarget data points for further analysis. Commonly used software for preprocessing includes CloudCompare, LASTools, and FUSION. Visualization techniques allow for evaluating data quality and provide insight into potential areas for further analysis. Postprocessing enables the creation of final products such as digital elevation models, contours, and canopy height models. This step typically involves using specialized software such as ArcGIS, QGIS, ENVI, Trimble eCognition, etc. LiDAR analysis involves using statistical and spatial analysis techniques to identify patterns and relationships in the data. This step is crucial for making informed decisions based on LiDAR data. LiDAR analysis methods vary depending on the application but may include clustering, regression analysis, and machine learning algorithms.
Conclusions
The use of LiDAR technology in the forestry sector has evolved over the decades. LiDAR applications are becoming more efficient and cheaper, increasing their potential in forestry. LiDAR technology has seen a surge in the forestry sector due to its ability to map the arrangement of forests, determine the size of forested areas, estimate biomass, identify changes, and assess potential forest hazards. This technology can aid forestry professionals in making more informed management decisions and developing plans by providing detailed, 3D information about their stands. However, obtaining LiDAR data can prove challenging, as some data may not be easily accessible or can be costly. Therefore, forest stakeholders need to consider the quality of LiDAR data, cost, available software for data analysis, data sources, and LiDAR platforms when incorporating this technology into their objectives. While LiDAR data acquisition may be more expensive than traditional aerial or satellite imagery, it can be more cost-effective than intensive conventional field data collection and provide more reliable estimates than other methods. Forestry professionals can use LiDAR data to gain detailed and high-quality information about their landscape by considering these factors.
References
- Brodu, N., & Lague, D. (2012). 3D terrestrial lidar data classification of complex natural scenes using a multi-scale dimensionality criterion: Applications in geomorphology. ISPRS Journal of Photogrammetry and Remote Sensing, 68, 121–134.
- Dassot, M., Constant, T., & Fournier, M. (2011). The Use of Terrestrial LiDAR Technology in Forest science: Application Fields, Benefits and Challenges. Annals of forest science, 68(5), 959-974.
- Dong, P., & Chen, Q. (2017). LiDAR remote sensing and applications. CRC Press.
- Felix, R. (2015). An Introduction to LiDAR. Independent publisher empowered by wordpress.
- Fernandez, J., Singhania, A., Caceres, J., Slatton, K., Starek, M., & Kumar, R. (2007). An overview of lidar point cloud processing software. GEM Center Report No. Rep_2007-12-001, University of Florida, 27.
- Puliti, S., Dash, J. P., Watt, M. S., Breidenbach, J., & Pearse, G. D. (2020). A comparison of UAV laser scanning, photogrammetry and airborne laser scanning for precision inventory of small-forest properties. Forestry: An International Journal of Forest Research, 93(1), 150–162.
- Shan, J., & Toth, C. K. (2018). Topographic laser ranging and scanning: principles and processing. CRC press.
- Wulder, M. A., White, J. C., Nelson, R. F., Næsset, E., Ørka, H. O., Coops, N. C., Hilker, T., Bater, C. W., & Gobakken, T. (2012). Lidar sampling for large-area forest characterization: A review. Remote Sensing of Environment, 121, 196–209.
- Young, A. P., Olsen, M., Driscoll, N., Flick, R., Gutierrez, R., Guza, R., Johnstone, E., & Kuester, F. (2010). Comparison of airborne and terrestrial lidar estimates of seacliff erosion in southern California. Photogrammetric engineering & remote sensing, 76(4), 421–427.
Arjun Rijal, Graduate Research Assistant; Richard Cristan, Extension Specialist, Assistant Professor; Manisha Parajuli, Graduate Research Assistant, all in Forestry, Wildlife, and Environment, Auburn University
New May 2023, Application of LiDAR in Forest Management, FOR-2138